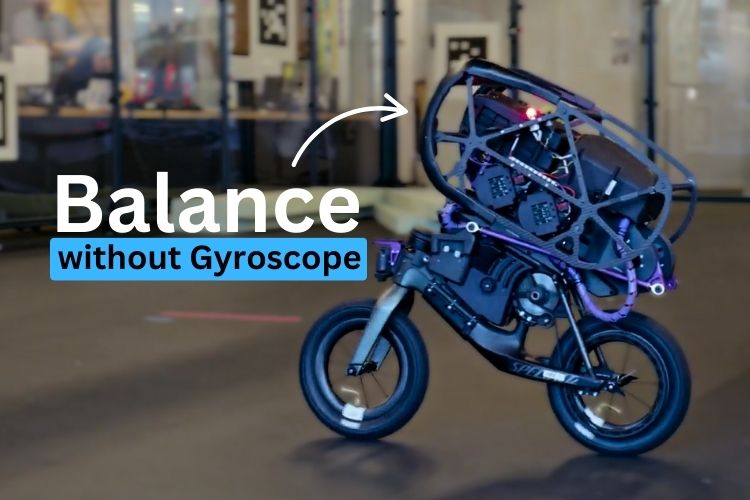
The Robotics and AI (RAI) Institute has developed the Ultra Mobility Vehicle (UMV), a self-balancing robotic bike capable of navigating challenging terrain and even jumping onto high surfaces. Reinforcement learning (RL) is playing a key role in helping robots adapt to complex situations and perform better.
Unlike traditional self-balancing bikes, this UMV does not use a gyroscope for stability. Instead, it moves like a regular bicycle, steering with its front wheel while shifting a weighted top section up and down to maintain balance. RL allows it to learn movements that would be difficult to achieve with conventional control methods.
One of the biggest challenges for the UMV is riding backward, which is highly unstable. Standard Model Predictive Control (MPC) struggles with this, especially on uneven ground. However, RL enables the robot to adapt and remain stable even in unpredictable conditions.
The UMV is first trained in a simulated environment before being tested in the real world. Simulation allows researchers to refine movement strategies quickly, though transferring these skills to physical robots remains a challenge. By integrating real-world data into simulations, researchers can improve accuracy and reliability.
As RL advances, it continues to redefine robotic movement. Whether it’s making a robotic bike jump onto a table or enhancing humanoid locomotion, RL is pushing the boundaries of robotics and automation.