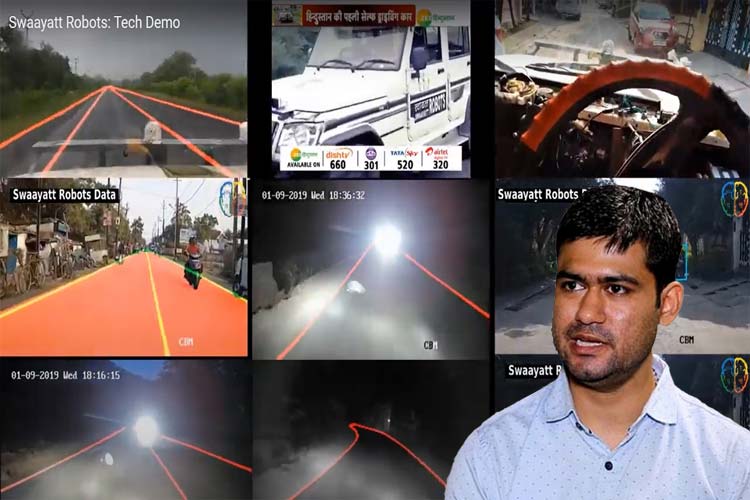
Tech giants like Tesla and Google have made self-driving vehicles the much talked about topic amongst tech enthusiasts. Various companies around the globe are working towards developing autonomous driving vehicles for various terrains.
To make connected autonomous driving technology accessible, affordable, and available to everyone, Bhopal-based Swaayatt Robots joined the bandwagon. However, with immense knowledge of all the technology involved in Autonomous Robotics, the CEO of the company, Mr. Sanjeev Sharma left many tech companies behind in the race. Since 2009, he has been researching a lot and undergoing mathematical calculations involved in coming up with smart solutions for self-driving cars.
We got the opportunity to talk to Mr. Sanjeev and know every bit of the technology behind Autonomous Vehicles and Robotics that Swaayatt Robots is working on and their future plans. Hit a jump to read on the entire conversation we had with him. Alternatively, you can also watch the video below to hear the conversation between our editor and Sanjeev himself
Q. Making Autonomous Driving Technology Accessible and Affordable to everyone is the main mission of Swaayatt Robots. How did the journey start?
I've been researching in the area of autonomous navigation for the past 11 years now. Back in 2009, I was inspired by the DARPA Grand Challenges that happened in the US. Autonomous driving became the goal of mine during those years. Over many years, I kept researching and did self-studies specifically on motion planning and decision making under uncertainties. The focus was on making optimum use of machine learning, reinforcement learning, and various techniques. I started Swaayatt Robots in 2014 but it was not simply applying the research and the studies that I had done over the past few years. Applying some ideas in motion and decision making, I had to solve the perception planning and localization problem as well. I had research experience only in the area of decision making and motion planning. But the areas of perception and localization were fairly new to me. My tremendous mathematical background helped me a lot.
Once I started developing the algorithmic frameworks to enable autonomous driving around 2015, I realized that this can be something very huge, and we can really solve the problem of autonomous driving in very stochastic adversarial traffic scenarios. And since 2014, I have been working full time on this startup. My research in particular covers several branches but, in particular, most of the focus of our company is to develop decision-making and motion planning algorithms that allow autonomous vehicles to deal with very high levels of stochasticity in traffic dynamics. That amounts to roughly 65% to 70% of the research that happens at Swaayatt Robots. Around 25% - 27% of the research goes into the area of perception, which encompasses all sorts of algorithms that process the sensor data from a vehicular robotic system, and build 3d representation of the world around it.
In perception, we are one of the very few companies in the world who can allow autonomous vehicles to perceive the environment using only off-the-shelf cameras that work during the day and night time too. This is roughly how the journey has been so far.
Q. You started in 2014 to validate your ideas and then you completely caught the path by 2015. So what should we do in this one year? How did you test that self-driving can be done in India?
Autonomous driving is the blend of three algorithmic pipelines put together viz. perception, planning, and localization. The algorithms take the sensory data, process it, and build a 3d representation around a vehicle. We call them perception algorithms. Localization algorithms try to globally accurately determine the position of the vehicle on road. This is how robots used to work in academic settings. In 2009, this model of autonomous driving was pioneered by Google. Before an autonomous vehicle navigates on a certain road, the entire road has to be mapped in very high detail in 3d. We call these maps, high fidelity maps. These high fidelity maps store some very key information about the environment. They typically store all the different kinds of delimiters in the environment.
Before the autonomous vehicle navigates in an environment, the entire environment is mapped in very precise manner. All the lane markers, road boundaries, and any kind of delimiter in the environment are actually stored in these kinds of high fidelity maps.
When the vehicle navigates through an environment for which you already have high-fidelity maps, then you again capture the data from various sensors on the vehicle and try to match the data with a reference map that you have built. This matching process gives you a pose vector that tells you where the vehicle is on planet earth and what is the configuration of the vehicle. Once you know the position and the configuration of the vehicle on road, the entire information that you had stored in the high-fidelity maps is projected on top of the vehicle's current configuration. When you project this information like road markers, lane markers, and any kind of road delimiter or environment delimiter; the autonomous vehicle knows where it is now with respect to a particular delimiter or from a particular lane marker. So, this is what localization algorithms do.
The final area of autonomous driving is planning and decision making. The more sophisticated and better the planning and decision-making algorithms you have, the more capable your autonomous vehicle is going to be. For example, planning and decision-making algorithms will differentiate companies from being at level two, level three, level four, and level five autonomy. Any algorithm responsible for decision making or planning the motion and behavior of the vehicle is a planning algorithm.
The more sophistication you have in the planning algorithms, the better your vehicle is going to be. Several motion planners and decision-makers help in evaluating the safety of the vehicle and the environment, the speed at which you are navigating, the surrounding of the vehicle, and all the parameters that you can compute from your environment. This is what planning algorithms do.
I've been researching in the area of planning. If you have the kind of algorithms that can deal with the stochasticity in the traffic dynamics in India. If you can deal with that and if you have algorithms, then you have proven that if you can just build a perception and localization stack, you have a full-fledged autonomous driving technology.
You don't need to develop all different algorithms to verify what works best. You just need to build three or four different algorithms that you know are going to solve the key problem in autonomous driving. Safety is the primary issue why you do not see commercial autonomous vehicles on road. Cost and all other issues are secondary. I could have built the entire startup on just one or two algorithms like the localization and mapping aspect of autonomous driving. But my goal was to develop a full-fledged autonomous vehicle and not one or two algorithms here and there. Having proven the key aspect in the area of planning and decision making gave me the confidence to tackle the entire problem of autonomous driving at large.
Q. What level of autonomous driving is Swaayatt Robots working on? And what level do you think is possible in India?
Our goal is to achieve level 5 autonomy and to ensure that the technology is safe in these kinds of environments. We are somewhere between level three and level four. Some of the algorithmic research that we're doing is in motion planning and decision making that is targeted towards level five.
We are also working on enabling autonomous vehicles to be able to cross the intersection at peak traffic hours without the traffic lights. We are targeting to achieve level-five autonomy by enabling autonomous vehicles in dealing with tight space with highly stochastic traffic. We have done autonomous driving in a very tight environment when a vehicle or a bike was coming from the opposite end as well. At the POC level, we have achieved between three and four-level. We have already turned the POCs for level-four autonomy by conducting experiments in highly stochastic traffic with tight spaces. Our current goal is to achieve 101 kilometers per hour autonomous driving on Indian roads.
Once you have proven the safety of the vehicle in these kinds of environments, you can take your technology and apply it anywhere else like in North America and Europe where the traffic is much more structured, where environments are also much stricter as compared to the Indian environments. So, India as of now is a testing ground for us to prove that we have something that no one else has done at the moment.
Q. How much has Swaayatt Robots progressed in developing an Autonomous Driving solution? What level of driving are you currently working on?
Currently, we have the world's fastest motion planning algorithm that can plan near-optimal time-parameterized trajectories for an autonomous vehicle in 500 microseconds. So the algorithm works roughly at 2000 hertz. We have the technology to enable up to 80 kilometers per hour autonomous driving on Indian highways. Achieving that kind of speed on Indian highways is very challenging. Typically, if you can do that, you can take it elsewhere as well. You can apply it in foreign traffic and basically, you're very close to level four. To give you an idea, we have been working on what we call multi-agent intent analysis and negotiation. This framework allows our vehicle to not just compute the probability of the intentions of other vehicles or agents on road. It can compute the probabilities of the entire path sets that other agents or vehicles or obstacles in the environment cannot. However, this capability alone is not sufficient. For example, you can build a very computationally demanding system that can predict the future motion trajectories and perhaps compute the probabilities of all the path sets of different vehicles. This is where you have to focus i.e. on the computational requirement as well. The computational demand in this problem of multi-agent intent analysis and negotiations will grow exponentially if you have not done any research, have not used the mathematics properly, or if you have not designed them properly. I'm researching some of the concepts from applied mathematics, specifically in the area of topological theory. I am using some of the concepts like homotopy maps, which allow our technology to scale the computations. At least as of now, it is superlinear in terms of the number of agents as opposed to the exponential blow-up that you would encounter if you have not worked out the mathematics behind the algorithms properly.
The multi-agent intent analysis negotiation framework is further subdivided into two different branches that we're currently working on. One is the TSN (Tight Space Negotiator Framework) and the other is the overtaking model. TSN allows the autonomous vehicles to negotiate both the tight environments and the stochastic traffic, both at low and high speeds. So high speed would be very useful for highway cluttered stochastic traffic scenarios and the low speed would be very useful when the vehicle is navigating in an urban scenario, where you often encounter the tightest streets with too much traffic and noise in the traffic which means there is too much uncertainty in the traffic dynamics.
We have already been working on this for the last two and a half years, and we have already developed it in the form of POC. Some of the bits and pieces of these frameworks that I'm talking about could be shown in the demo in our next experiment which will be targeted towards achieving 101 kilometers per hour functioning on Indian roads.
Furthermore, we have also been researching in different branches of AI. We heavily use apprenticeship learning, inverse reinforcement learning. So, we are currently working on enabling autonomous vehicles to overtake on typical two-lane roads just like Indian drivers do. We are proving both in simulation as well as in the real-world to the maximum extent possible with limited funding. These are some of the research areas that we have already proven on the ground, and some of them are going to be proven in the next few months.
Apart from that, we are one of the only companies in the world that can enable autonomous driving in completely unknown and unseen environments for which there are no high-fidelity maps at all. We can enable autonomous driving without the use of high-fidelity maps. We are in the business of completely eradicating the need for high fidelity maps and this eradication is enabled by two of our key technologies. Our TSN framework is made to set a new regulatory benchmark.
Q. Speaking about the hardware architecture, what kind of hardware do you use for your computational purpose. Also, what kind of sensors and cameras do you use to map the real world on your autonomous vehicles?
As of now, we just use off-the-shelf cameras. If you see our demo for an autonomous vehicle, you'll notice that we had used nothing more than a 3000 Rs camera. If you look at the perception research that is happening all over the world with the autonomous companies or robotics companies for that matter, they're using all three different sensors like cameras, LiDARs, and radars. Currently, all our autonomous driving experiments have happened only using cameras. When I began the company, I only had expertise in planning but since 2016, I realized that state-of-the-art research papers whatever the labs all over the world are working on; it just doesn't work in the real world. If they work, they're too computationally intensive, and they just do not work. So, I took perception as my primary research area as well and I devoted around 25% - 27% of my time in doing perception research. Now, the research goal of our company is to enable autonomous vehicles to be able to perceive using only the cameras without the need for LiDARs and radars. This is a research ambition that we want to achieve. While achieving that, we have also ensured that we have the world's fastest algorithm for any common task.
We have two goals in perception. One, the algorithm should be so capable that they enable autonomous vehicles to perceive using only cameras both during day and night. We have extended this perception capability not just for the day time but at night as well using nothing but the headlight of the vehicle and regular off-the-shelf RGB and NIR cameras, the kind of cameras that you can buy for 3000 Rs in the market.
We focus more on accuracy and speed. We've also focused on the computational requirement. So our semantic simulation framework is up to 30 times faster than what the state of the art can offer you and significantly faster than what other technology supply company can offer you.
We've also been researching on one more area called delimiters prediction. Lane markers and road boundaries are salined delimiters and obvious to notice. There are also non-salined delimiters in the environment that are hard to detect. Typically, it's very hard to develop algorithms that can almost always detect delimiters with a 100% guarantee. This is the primary reason why autonomous vehicle companies use a high-fidelity map as a backup. But the problem is that the process of extraction of data from high fidelity maps is so complex that the computations to localize a vehicle against HFMs cannot be done in real-time using onboard computers. So, you need to have internet connectivity.
Delimiter prediction is our very broad research area. The key aspect of our framework is that it both detects lane markers and road delimiters in case if they are visible. If they're not present in the environment or visible in images, it automatically generates them in real-time. This allows us to get rid of the high-fidelity map combined with our planning technology.
In terms of the perception algorithm capability, we can detect and generate. We developed a free space direction algorithm called Deep Energy Maps in 2017, and we are far ahead of what other companies offer. Our Deep Energy Maps work in any kind of scenario and it can actually form the non-complex bounding box around obstacles. Unlike other companies’ framework, our system is 10 times faster than what other companies like what NVIDIA has developed.
Q. Swaayatt Robots uses cameras to operate the vehicles even during evening or nighttime. Is it safe to assume that you will never be looking into LiDAR or Radar for your algorithms? Or do you have plans to incorporate them as well?
When I started the company, LiDARs were very expensive, and importing them to India would cost 80-85 lakhs. In comparison to these, the cameras were quite affordable. All that is required in the autonomous vehicles field is proper mathematical formulations. I had developed formulations to enable autonomous vehicles to perceive using just cameras. The algorithmic ideas that we have developed for processing the camera data, the same ideas directly extended to LiDAR as well. For example, one of the state-of-the-art algorithms was published last year, it's called range net + +. It is used to segment LiDAR data. We extended our algorithm for the idea of LiDAR data segmentation. So, there's a deep geometric network framework that we've developed to semantically segment images. The same idea that we used in the deep geometric network for cameras, we extended it to LiDAR and found that we are 11 times faster than the range net ++ algorithm which is used to process LiDAR data.
Furthermore, we are looking at potential collaboration with a few of the LiDAR companies as well to develop algorithmic solutions. “Going forward, if there's no collaboration with any of the LiDAR companies, we will ultimately make the LiDARs redundant. LiDAR provides you a data that adds another information dimension to your entire data that you would gather just from the cameras. For example, they provided the 3d representation of the world directly without much effort. And we can use that to make our algorithms more robust.
We have developed an algorithm for perceiving at night. If we add 3d structural information on top of the camera data, we can do much more. Any algorithm that has been developed to process the data, at least in our company, the idea is extended to the LiDAR data processing as well.
Q. How much of trials and testing has Swaayatt Robots performed on Indian Roads? What are your major findings and observations?
We have around 550 plus million images that we have collected from autonomous systems. We have a local cluster of computers that we use to train our systems and the process is entirely mathematical in nature. In Deep Energy Maps, the loss function of the neural network is related to the flow of energy in physics and for us, the challenge was to model that as the loss function in a network and relate it with the pixels. So, the process is entirely mathematical, you have to study various mathematical branches to come up with the ideas. If we talk about planning, I have several ideas for which we do not have enough funding to execute but these can reduce redundancy in autonomous driving, ability to work without high fidelity maps is just one of those. Similarly, in semantic segmentation, we have the fastest block of semantic segmentation. The question here is how did we achieve that? It is not just the implementation trick; we have to do a lot of mathematical research.
We have developed a new type of computational unit for the neural network. I call it the geometric compute unit that I developed a few years back and this unit allows us to perform lesser computation and extract more semantic information from the images. This is how we developed the world’s fastest network for semantic segmentation. We are also working on various collaborations and in the future, we will be able to make implementations.
Q. How do you see the market for Autonomous driving in India? What are the immediate applications for these technologies?
Autonomous driving in India would take some time. In India, autonomous driving has key usage in military operations. We are surrounded by adversaries and a new adversary is also coming into the picture. The defense is one area where autonomous driving will find tremendous use whether our country realizes this at the moment or not. There is enough focus on that. Besides, the government is undertaking several initiatives to upgrade defense capabilities. Therefore, more and more prominent applications of robotics and autonomous driving will find use in different scenarios. Apart from that, autonomous driving will come to the Indian market first in the form of ADAS because ADAS doesn’t have to take control of the vehicle. This is one of the diluted versions of technology at large. By 2025, the ADAS market will be a 36 billion dollar market in India. We don’t have to create the market; the market already exists.
Q. What are your plans and targets when can we expect Swaayatt Robots to be market-ready?
We have already done the demo of our technology in a proof of concept manner. We have collaborated with various OEMs in Europe. Apart from that, we have collaborated with some of the LiDAR companies in the US. We are looking into collaborating with companies that have got C level funding. We provide our technology both in an end-to-end fashion and as modular solutions. We are talking to one of our potential clients in industrial robotics for integrating our technology. These are some areas that we are targeting as of now, and we plan to expand our horizon in the years to come.