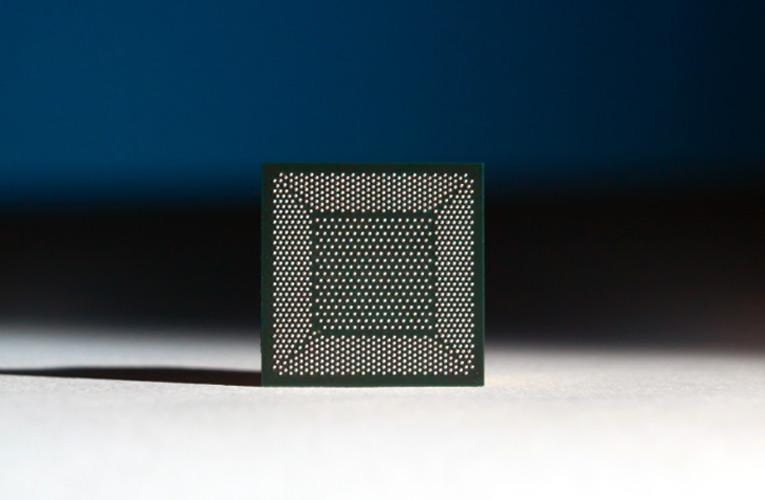
Researchers from Intel Labs and Cornell University have demonstrated the unique ability of Intel’s neuromorphic research chip named Loihi to learn and identify hazardous chemicals. The research was published in the journal Nature Machine Intelligence describing how a neural algorithm was built from scratch based on the architecture and dynamics of the human brain’s olfactory circuits.
The chip is based on a neuromorphic computing architecture that’s inspired by scientists’ current understanding of the human brain and how it solves problems. It is a bit of hardware that aims to mimic how the human brain processes and solves problems. It can leverage the knowledge that it already possesses to make inferences about new data, thereby helping to speed up its learning process exponentially over time.
The chip has the ability to identify each chemical based on its odor from just a single test sample that too without disrupting its memory of previously learned scents. As compared to any conventional recognition system like a deep learning system that requires around 3,000 times more training samples to reach the same level of accuracy, the chip works with superior accuracy.
It can learn and recognize the scent of 10 different hazardous chemicals. The Intel team used a dataset that consists of the activity of 72 known chemical sensors in the brain and how they respond to the smell of each chemical. The data were further used to configure what the team calls “a circuit diagram of biological olfaction” on Loihi. With this, Loihi could recognize the neural representation of each smell and identify each one, even with significant occlusion.
Loihi’s olfactory capabilities could be put to use on new electronic nose systems that help doctors to diagnose diseases. Moreover, it can be used to develop systems for detecting weapons and explosives at airports. It could also be used to develop effective smoke and carbon monoxide detectors. From sensory scene analysis (understanding the relationships between objects you observe) to abstract problems like planning and decision-making, the researchers further plan to generalize this approach to a wider range of problems.