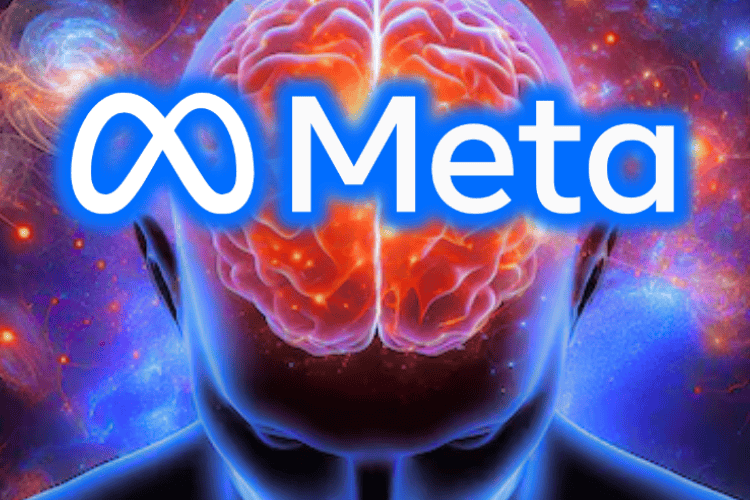
Meta AI has introduced Brain2Qwerty, a new artificial intelligence system that converts brain signals into text without the need for surgery. The system uses non-invasive methods including electroencephalography (EEG) and magnetoencephalography (MEG) to record brain activity. In the study, healthy volunteers memorized sentences and then typed them on a QWERTY keyboard while their brain signals were captured. This innovative approach may help people who have lost the ability to speak or move, offering a safer alternative to invasive neuroprostheses that require brain surgery.
Brain2Qwerty works in three simple steps. First, a convolutional module examines short 500-millisecond segments of brain activity to find useful patterns. Next, a transformer module processes the sequence of signals to understand the context of the typed sentence. Finally, a pre-trained language model refines the text output by correcting errors. The study involved recordings from 35 participants. Results show that when using MEG, the system achieved an average character error rate of 32% with the best cases reaching just 19%. In comparison, EEG recordings resulted in a 67% error rate.
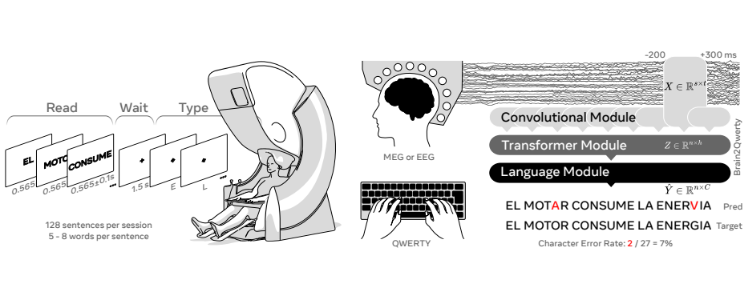
Researchers compared Brain2Qwerty with other common models such as a linear classifier and EEGNet and found that Brain2Qwerty provided better performance in decoding both hand movements and characters. The higher quality of MEG signals with a better signal-to-noise ratio than EEG contributed to this improved accuracy. These findings demonstrate that advanced deep learning methods can enhance non-invasive brain-computer interfaces, making them more reliable and closer in performance to invasive systems.
This breakthrough is a significant step in brain-computer interface research. However, challenges remain as MEG devices are expensive and require specialized facilities. Meta is exploring newer, more affordable technologies to make this system accessible in the future. Additionally, the study was conducted on healthy individuals and further research is needed to adapt it for people with severe disabilities. Meta AI’s work on Brain2Qwerty offers hope for safer communication methods for patients with speech or movement difficulties. The study highlights how combining deep learning with advanced brain recording techniques can lead to practical healthcare solutions.