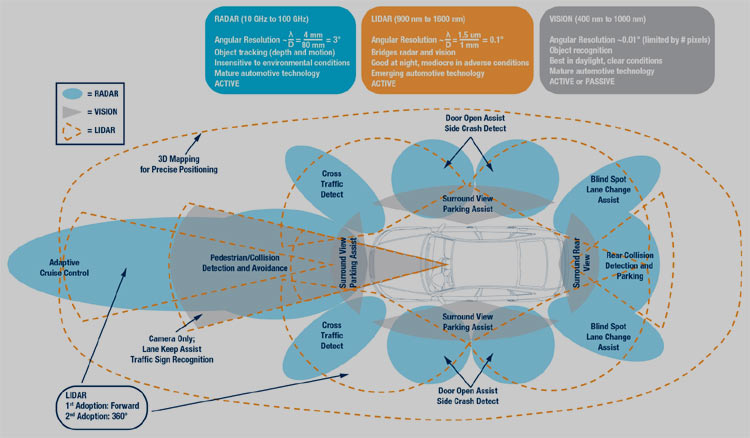
It’s the moonshot of our time. From sensors to artificial intelligence (AI), the classic electronics supply chain has formed a collaborative matrix dedicated to making autonomous vehicles safe. To that end, there is much to be done in hardware and software development to ensure drivers, passengers, and pedestrians are protected. While machine learning and AI have a role to play, their effectiveness depends upon the quality of the incoming data. As such, no autonomous vehicle can be considered safe unless it is built upon a foundation of high performance, high integrity sensor signal chains, to consistently supply the most accurate data upon which to base life or death decisions.
Like the original moonshot, there are many obstacles on the road to safe autonomous vehicles. Recent high profile incidents involving self-driving vehicles feed into the nay-sayer narrative that vehicles and the environments in which they operate are too complex, there are too many variables, and the algorithms and software are still too buggy. For anyone who has been involved with compliance testing to ISO 26262 Functional Vehicle Safety, they’d be forgiven for being skeptical. And that skepticism is supported by charts comparing the number of physical miles driven to the number of disengagements from the autonomous mode for five autonomous vehicle companies testing in Silicon Valley in 2017 (Figure 1). Figures for 2019 have yet to be compiled, but reports for individual companies are available online.
However, the goal has been set and the imperative is clear: vehicle autonomy is coming, and safety is paramount. The unofficial 2018 California autonomous vehicle Department of Motor Vehicles (DMV) numbers show that the number of disengagements per mile is decreasing, which also shows that the systems are getting more capable. However, this trend needs to be accelerated.
Putting collaboration and new thinking first, automotive manufacturers are talking directly to silicon vendors; sensor makers are discussing sensor fusion with AI algorithm developers; software developers are finally connecting with hardware providers to get the best out of both. Old relationships are changing, and new ones are forming dynamically to optimize the combination of performance, functionality, reliability, cost, and safety in the final design.
End to end, the ecosystem is pursuing the right models upon which to build and test fully autonomous vehicles for quickly emerging applications such as Robo-taxis and long-haul trucking. Along the way, higher degrees of automation are being achieved rapidly as a result of improvements in sensors that push the state of the art in advanced driver assistance systems (ADAS).
These sensor technologies include cameras, light detection and ranging (lidar), radio detection and ranging (radar), microelectromechanical systems (MEMS), inertial measurement units (IMUs), ultrasound, and GPS, which all provide the critical inputs for AI systems that will drive the truly cognitive autonomous vehicle.
Cognitive Vehicles Foundational to Predictive Safety
Vehicle intelligence is often expressed as levels of autonomy. Level 1 (L1) and L2 are largely warning systems, while a vehicle at L3 or greater is empowered to take action to avoid an accident. As the vehicle progresses to L5, the steering wheel is removed and the car operates fully autonomously.
In these first few system generations, as vehicles start to take on L2 functionality, the sensor systems operate independently. These warning systems have a high false alarm rate and are often turned off since they are a nuisance.
To achieve fully cognitive autonomous vehicles, the number of sensors increases significantly. Additionally, their performance and response times must greatly improve (Figure 3, Figure 4).
With more sensors built into vehicles, they can also better monitor and factor in current mechanical conditions, such as tire pressure, change in weight (for example, loaded vs. unloaded, one passenger or six), as well as other wear and tear factors that might affect braking and handling. With more external sensing modalities, the vehicle can become more fully cognitive of its health and surroundings.
Advances in sensing modalities allow an automobile to recognize the current state of the environment and also be aware of its history. This is due to the principles developed by Dr. Joseph Motola, chief technologist in the
ENSCO Aerospace Sciences and Engineering Division. This sensing ability can be as simple as awareness of road conditions, such as the location of potholes, or as detailed as the types of accidents and how they occurred in a certain area over time.
At the time these cognitive concepts were developed, the level of sensing, processing, memory capacity, and connectivity made them seem far-fetched, but much has changed. Now, this historical data can be accessed and factored into real-time data from the vehicle’s sensors to provide increasingly accurate degrees of preventive action and incident avoidance.
For example, an IMU can detect a sudden bump or swerve indicating a pothole or an obstacle. In the past, there was nowhere to go with this information, but real-time connectivity now allows this data to be sent to a central database and used to warn other vehicles of the hole or obstacle. The same is true for the camera, radar, lidar, and other sensor data.
This data is compiled, analyzed, and fused so that it can inform the vehicle’s forward-looking comprehension of the environment in which it operates. This allows the vehicle to act as a learning machine that will potentially
make better, safer decisions than a human can.
Multifaceted Decision Making and Analysis
Much progress has been made in advancing state-of-the-art vehicle perception. The emphasis is on gathering the data from the various sensors and applying sensor fusion strategies to maximize their complementary strengths, and support their respective weaknesses, under various conditions (Figure 5).
Still, much has yet to be done if they are to be truly viable solutions for the problems the industry faces. For example, cameras can calculate lateral velocity (that is, the speed of an object traveling orthogonally to the direction of travel of the vehicle). Still, even the best machine learning algorithms require ~300 ms to make a lateral movement detection with sufficiently low false alarm rates. For a pedestrian moving in front of a vehicle moving at 60 mph, milliseconds can make the difference between superficial and life-threatening injuries, so response time is critical.
The 300 ms delay is due to the time required to perform delta vector calculations from successive video frames. Ten or more successive frames are required for reliable detection: we must get this down to one or two successive frames to give the vehicle time to respond. Radar has the capability to achieve this.
Similarly, radar has many advantages for speed and object detection, such as high resolution in both azimuth and elevation, as well as the ability to “see” around objects, but it too needs to provide more time for the vehicle to react. With a goal of 400 km/hour or greater unambiguous velocity determination, new developments in 77 GHz to 79 GHz operation are making headway. This level of velocity determination may seem extreme but is necessary to support complex divided highway use cases where vehicles are traveling in opposite directions at speeds in excess of 200 km/hour.
Bridging cameras and radar is lidar, the characteristics of which have made it a viable and essential element of the fully cognitive vehicle (Figure 6). But it too has challenges that need to be overcome going forward.
Lidar is evolving into compact, cost-effective solid-state designs that can be placed at multiple points around the vehicle to support full 360˚ cover- age. It complements radar and camera systems, adding higher angular resolution and depth perception to provide a more accurate 3D map of the environment.
However, its operation at near-infrared (IR) (850 nm to 940 nm) can be harmful to the retina so its energy output is tightly regulated to 200 nJ per pulse at 905 nm. However, by migrating to shortwave IR, at over 1500 nm, the light is absorbed over the entire surface of the eye. This allows more relaxed regulatory requirements of 8 mJ per pulse. At 40,000 times the energy level of 905 nm lidar, 1500 nm pulsed lidar systems provide 4× longer range. Also, 1500 nm systems can be more robust against certain environmental conditions, such as haze, dust, and fine aerosols.
The challenge with 1500 nm lidar is system cost, which is largely driven by the photodetector technology (which today is based on InGaAs technology). Getting to a high-quality solution—with high sensitivity, low dark current, and low capacitance—is the key enabler for the 1500 nm lidar. Additionally, as lidar systems progress into generation 2 and 3, application-optimized circuit integration will be needed to drive size, power, and overall system costs down.
Beyond ultrasound, cameras, radar, and lidar, there are other sensing modalities that have critical roles to play in enabling fully cognitive autonomous transportation. GPS lets a vehicle know where it is at all times. That said, there are places where GPS signals are not available, such as in tunnels and among high rise buildings. This is where inertial measurement units can play a critical role.
Though often overlooked, IMUs depend upon gravity, which is constant, regardless of environmental conditions. As such, they are very useful for dead reckoning. In the temporary absence of a GPS signal, dead reckoning uses data from sources such as the speedometer and IMUs to detect distance and direction traveled and overlays this data onto high definition maps. This keeps a cognitive vehicle on the right trajectory until a GPS signal can be recovered.
High-Quality Data Saves Time and Lives
As important as these sensing modalities may be, none of these critical sensor inputs matter if the sensors themselves are not reliable and if their output signals are not captured accurately to be fed upstream as high precision sensor data: the phrase “garbage in, garbage out,” has rarely held so much import.
To achieve this, even the most advanced analog signal chains must be continuously improved to detect, acquire, and digitize sensor signal outputs so that their accuracy and precision do not drift with time and temperature. With the right components and design best practices, the effects of notoriously difficult issues such as bias drift with temperature, phase noise, interference, and other instability-causing phenomena can be greatly mitigated. High precision/high-quality data is fundamental to the ability of machine learning and AI processors to be properly trained and to make the right decisions when putting into operation. And there are few second chances.
Once the data’s quality is assured, the various sensor fusion approaches and AI algorithms can respond optimally toward a positive outcome. It’s simply a fact that no matter how well an AI algorithm is trained, once the model is compiled and deployed on devices at the network edge, they are completely dependent upon reliable, high precision sensor data for their efficacy.
This interplay between the sensor modalities, sensor fusion, signal processing, and AI has profound effects upon both the advancement of smart, cognitive, autonomous vehicles and the confidence with which we can ensure the safety of drivers, passengers, and pedestrians. However, all is moot without highly reliable, accurate, high precision sensor information, which is so foundational to safe autonomous vehicles.
As with any advanced technology, the more we work on this, the more complex use cases are identified that need to be addressed. This complexity will continue to confound existing technology, so we need to look forward to next-generation sensors and sensor fusion algorithms to address these issues.
Like the original moonshot, there is an aspiration that the entire initiative of autonomous vehicles will have a transformative and long-lasting impact on society. Moving from driver assistance to driver replacement will not only improve the safety of transportation dramatically, but it will also lead to huge productivity increases. This future all rests on the sensor foundation upon which everything else is built.
Analog Devices has been involved in automotive safety and ADAS for the past 25 years. Now ADI is laying the groundwork for an autonomous tomorrow. Organized around centers of excellence in inertial navigation and monitoring and high-performance radar and lidar, Analog Devices offers high-performance sensor and signal/power chain solutions that will not only dramatically improve the performance of these systems but also reduce the total cost of ownership of the entire platform—accelerating our pace into tomorrow.
About the Author
Chris Jacobs joined ADI in 1995. During his tenure at Analog Devices, Chris has held a number of design engineering, design management, and business leadership positions in the consumer, communications, industrial, and automotive teams. Chris is currently the vice president of the Autonomous Transportation and Automotive Safety business unit at Analog Devices. Prior to this, Chris was the general manager of automotive safety, product and technology director of precision converters and the product line director of high-speed converters and isolation products.
Chris has a Bachelor of Science in computer engineering from Clarkson University, a Master of Science in electrical engineering from Northeastern University, and a Master of Business Administration from Boston College. He can be reached at chris.jacobs@analog.com.